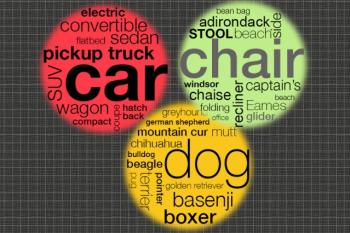
Christine Daniloff/MIT
Researchers from MIT’s Computer Science and Artificial Intelligence Laboratory and its Laboratory for Information and Decision Systems have designed a new algorithm that makes it much more practical to select diverse subsets from a much larger dataset.
Article Author
Date Published
December 16, 2016
Link to Original Article
When data sets get too big, sometimes the only way to do anything useful with them is to extract much smaller subsets and analyze those instead.
Those subsets have to preserve certain properties of the full sets, however, and one property that’s useful in a wide range of applications is diversity. If, for instance, you’re using your data to train a machine-learning system, you want to make sure that the subset you select represents the full range of cases that the system will have to confront.
Last week at the Conference on Neural Information Processing Systems, researchers from MIT’s Computer Science and Artificial Intelligence Laboratory and its Laboratory for Information and Decision Systems presented a new algorithm that makes the selection of diverse subsets much more practical.
With standard algorithms, selecting a subset of data points from a set with, say, a million data points would have been, effectively, impossible on a desktop computer. With the researchers’ new algorithm, it would take minutes.
“We want to pick sets that are diverse,” says Stefanie Jegelka, the X-Window Consortium Career Development Assistant Professor in MIT’s Department of Electrical Engineering and Computer Science and senior author on the new paper. “Why is this useful? One example is recommendation. If you recommend books or movies to someone, you maybe want to have a diverse set of items, rather than 10 little variations on the same thing. Or if you search for, say, the word ‘Washington.’ There’s many different meanings that this word can have, and you maybe want to show a few different ones. Or if you have a large data set and you want to explore — say, a large collection of images or health records — and you want a brief synopsis of your data, you want something that is diverse, that captures all the directions of variation of the data.
“The other application where we actually use this thing is in large-scale learning. You have a large data set again, and you want to pick a small part of it from which you can learn very well.”
Joining Jegelka on the paper are first author Chengtao Li, a graduate student in electrical engineering and computer science; and Suvrit Sra, a principal research scientist at MIT’s Laboratory for Information and Decision Systems.
Thinking small
Traditionally, if you want to extract a diverse subset from a large data set, the first step is to create a similarity matrix — a huge table that maps every point in the data set against every other point. The intersection of the row representing one data item and the column representing another contains the points’ similarity score on some standard measure.
There are several standard methods to extract diverse subsets, but they all involve operations performed on the matrix as a whole. With a data set with a million data points — and a million-by-million similarity matrix — this is prohibitively time consuming.
The MIT researchers’ algorithm begins, instead, with a small subset of the data, chosen at random. Then it picks one point inside the subset and one point outside it and randomly selects one of three simple operations: swapping the points, adding the point outside the subset to the subset, or deleting the point inside the subset.
The probability with which the algorithm selects one of those operations depends on both the size of the full data set and the size of the subset, so it changes slightly with every addition or deletion. But the algorithm doesn’t necessarily perform the operation it selects.
Again, the decision to perform the operation or not is probabilistic, but here the probability depends on the improvement in diversity that the operation affords. For additions and deletions, the decision also depends on the size of the subset relative to that of the original data set. That is, as the subset grows, it becomes harder to add new points unless they improve diversity dramatically.
This process repeats until the subset is adequately diverse; the question is how many repetitions are enough. In general, this is a difficult question, but the MIT researchers were able to answer it, and to prove that the answer will always be reasonable.
Making recommendations
“The most practically useful part of this work, so far, is for the recommendation problem,” says Le Song, an assistant professor of computational science and engineering at Georgia Tech. “The model — a so-called determinantal point process — is a really elegant model for this type of problem. But this model previously hasn’t been practical. People came up with all kinds of approximations for the problem, but none of these approximations is as elegant as Stefanie’s work.”
“But you can imagine another scenario where, for instance, you have a long document, and you want to take the five sentences that best summarize it,” Song adds. “Stephanie’s method can also do that. Or if you have a video and you want to generate a trailer, then you need to take some frames of the video and put them together. You want these frames to be representative of the whole video, while at the same time, they’re not always the same thing. You want to see differences.”
Reprinted with permission of MIT News.