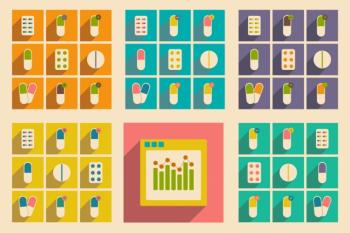
Article Author
Date Published
September 17, 2015
Link to Original Article
Clinical trials of new drugs or devices face a problem that most empirical inquiries don’t: They must not only provide clear data about toxicity and efficacy but also try to maximize the quality of treatment for all of the patients enrolled.
Online advertisers face a similar quandary. They want to test variants of ads to see which drive more traffic, but they also want the better-performing ads to reach more viewers.
In a forthcoming paper in the Annals of Statistics, Philippe Rigollet, an assistant professor of mathematics at MIT, and colleagues present a new way to organize such tests that’s mathematically guaranteed to yield the optimal result. Rigollet believes that the work could also have implications for the distribution of tasks in parallel computers.
“It’s called the ‘exploration-versus-exploitation dilemma,’” Rigollet says. “It was a bit of a dormant field in the ’80s and ’90s, and then in the early 2000s came Amazon and Google, and they had exactly this problem. In this paper, we looked at a variation of this thing that nobody, for some odd reason, had looked at.”
In principle, the best way to resolve the exploration-versus-exploitation dilemma for a given group of test subjects is to test one of them at a time. The first few subjects’ reactions to either of two drugs, for instance, could guide the treatment of the subsequent subjects. If, in the early going, one drug was clearly outperforming the other, it would be administered to the rest of the subjects, meeting the physician’s obligation to provide them with the best available treatment.
Of course, in a clinical trial, that approach would be woefully impractical, since it could take a year or more to determine each subject’s response to a new treatment. So clinical trials instead group subjects into batches. “People have started batching on the Internet as well, because it’s just too much to process,” Rigollet says. “There are so many people going on Google every second, they cannot wait to see what everybody’s doing.”
Few regrets
Rigollet and his collaborators — Vianney Perchet of the Université Paris Diderot, Sylvain Chassang of Princeton University, and Erik Snowberg of Caltech — show that with their technique, three or four batches of tests will generally yield results that are as good as those of testing subjects individually.
The measure of effectiveness they use is called “cumulative regret,” or the aggregate difference between the rewards that the subjects in the trial received — in the case of a drug trial, the mitigation of disease symptoms — and the rewards that they would have received had they all been administered what proved to be the best-performing option.
With a trial that tests subjects individually, the cumulative regret is proportional to the square root of the number of test subjects, or N0.5. With the researchers’ technique, four batches will at worst yield a cumulative regret proportional to N0.53, and three batches N0.57.
Those figures, however, assume that N can grow arbitrarily large. If it’s less than a million — which is almost always the case in clinical trials — then, in fact, just three batches will yield a cumulative regret proportional to N0.5. As the U.S. Food and Drug Administration currently requires four rounds of trials for new drugs, adopting the researchers’ scheme would not be a major disruption.
Sizing up probabilities
Moreover, Rigollet and his colleagues offer a principled way to determine the size of each batch. “The FDA requires four batches, but the number of people you have to take in each batch is not very well defined,” Rigollet says. “What our rule gives us is a way to say, ‘If you give me the number of patients you want to see, I can give you exactly the sizes of the batches you need to use.’”
The researchers’ approach is simple: After each batch, they calculate the uncertainty of its results. That uncertainty can be thought of as error bars around an average value. After the first phase of a clinical trial, for instance, 10 patients who took a new hepatitis drug for four weeks might have seen the virus count in their bloodstreams go down by an average of 50 percent. But statistically, because the sample size is so small, the true average for the population at large could be anywhere between 40 and 60 percent. Those values define the endpoints of the error bars.
In a trial comparing two drugs, if the error bars still overlap after any given round, both drugs continue into the next round; if they don’t, only the better-performing one continues. The same approach works for tests comparing more than two alternatives. Only those whose error bars overlap with the top-performing candidate continue from one round to the next.
For the researchers, the hard work wasn’t devising this protocol but proving its optimality — and determining how to size the batches. In ongoing work, they’re investigating trials that determine how different populations respond to different alternatives. It could be, for instance, that 20-year-old males and 50-year-old females would respond well to different ads for the same product.
The type of problem that Rigollet and his colleagues address is called a “bandit problem,” after “one-armed bandit,” a euphemism for slot machines. Someone trying to determine which of several slot machines offers the best rate of return without going broke in the process faces the exploration-versus-exploitation dilemma.
“This new result exhibits algorithms that adjust treatments only a very small number of times,” says Moritz Hardt, a research scientist at Google. “Surprisingly, as Rigollet and his co-authors elegantly prove, these algorithms still find a treatment that is nearly as good as the best single treatment in hindsight. This new development holds the promise of making bandit optimization a more robust choice across several application domains.”
Sébastien Bubeck, a researcher in the Theory Group at Microsoft Research, agrees. “There is little doubt that this new work will have an impact on how bandit algorithms are used in practice,” he says.
Rigollet’s work was funded by the National Science Foundation.
Reprinted with permission of MIT News.