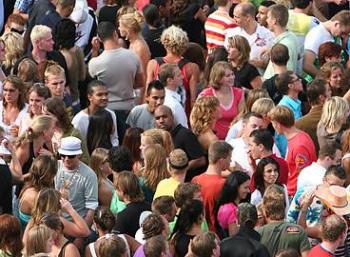
Article Author
Date Published
November 16, 2010
Link to Original Article
The rise of the Internet has sparked a fascination with what The New Yorker’s financial writer James Surowiecki called, in a book of the same name, “the wisdom of crowds”: the idea that aggregating or averaging the imperfect, distributed knowledge of a large group of people can often yield better information than canvassing expert opinion.
But as Surowiecki himself, and many commentators on his book, have pointed out, circumstances can conspire to undermine the wisdom of crowds. In particular, if a handful of people in a population exert an excessive influence on those around them, a “herding” instinct can kick in, and people will rally around an idea that could turn out to be wrong.
Fortunately, in a paper to be published in the Review of Economic Studies, researchers from MIT’s Departments of Economics and Electrical Engineering and Computer Science have demonstrated that, as networks of people grow larger, they’ll usually tend to converge on an accurate understanding of information distributed among them, even if individual members of the network can observe only their nearby neighbors. A few opinionated people with large audiences can slow that convergence, but in the long run, they’re unlikely to stop it.
In the past, economists trying to model the propagation of information through a population would allow any given member of the population to observe the decisions of all the other members, or of a random sampling of them. That made the models easier to deal with mathematically, but it also made them less representative of the real world. “What this paper does is add the important component that this process is typically happening in a social network where you can’t observe what everyone has done, nor can you randomly sample the population to find out what a random sample has done, but rather you see what your particular friends in the network have done,” says Jon Kleinberg, Tisch University Professor in the Cornell University Department of Computer Science, who was not involved in the research. “That introduces a much more complex structure to the problem, but arguably one that’s representative of what typically happens in real settings.”
Groupthink
Earlier models, Kleinberg explains, indicated the danger of what economists call information cascades. “If you have a few crucial ingredients — namely, that people are making decisions in order, that they can observe the past actions of other people but they can’t know what those people actually knew — then you have the potential for information cascades to occur, in which large groups of people abandon whatever private information they have and actually, for perfectly rational reasons, follow the crowd,” Kleinberg says. The MIT researchers’ paper, however, suggests that the danger of information cascades may not be as dire as it previously seemed.
Ilan Lobel, who got his PhD last year from MIT’s interdepartmental Operations Research Center and now teaches at New York University, and his three thesis advisors, Daron Acemoglu of economics and Munther A. (“Munzer”) Dahleh and Asuman Ozdaglar of the Laboratory for Information and Decision Systems (LIDS), developed a mathematical model that describes attempts by members of a social network to make binary decisions — such as which of two brands of cell phone to buy — on the basis of decisions made by their neighbors. The model assumes that for all members of the population, there is a single right decision: one of the cell phones is intrinsically better than the other. But some members of the network have bad information about which is which.
The MIT researchers analyzed the propagation of information under two different conditions. In one case, there’s a cap on how much any one person can know about the state of the world: even if one cell phone is intrinsically better than the other, no one can determine that with 100 percent certainty. In the other case, there’s no such cap. There’s debate among economists and information theorists about which of these two conditions better reflects reality, and Kleinberg suggests that the answer may vary depending on the type of information propagating through the network. But previous models had suggested that, if there is a cap, information cascades are almost inevitable.
The MIT researchers showed that if there’s no cap on certainty, an expanding social network will eventually converge on an accurate representation of the state of the world; that wasn’t a big surprise. But they also showed that in many common types of networks, even if there is a cap on certainty, convergence will still occur.
“We are not the first ones to look at this problem, but people in the past have looked at it using more myopic models,” says Acemoglu. “They would be averaging type of models: so my opinion is an average of the opinions of my neighbors’.” In such a model, Acemoglu says, the views of people who are “oversampled” — who are connected with a large enough number of other people — will end up distorting the conclusions of the group as a whole. “What we’re doing is looking at it in a much more game-theoretic manner, where individuals are realizing where the information comes from. So there will be some correction factor,” Acemoglu says. “If I’m seeing you, your action, and I’m seeing Munzer’s action, and I also know that there is some probability that you might have observed Munzer, then I discount his opinion appropriately, because I know that I don’t want to overweight it. And that’s the reason why, even though you have these influential agents — it might be that Munzer is everywhere, and everybody observes him — that still doesn’t create a herd on his opinion.”
All about me
At first blush, the involvement of computer scientists in the project might seem a little odd, but as Dahleh, the acting director of LIDS, explains, “The engineering literature tackled almost the same problem, but the agents were not selfish. The idea is trying to design the network so that the convergence can happen as quickly as possible.” For instance, he says, in a network of distributed sensors, the engineering challenge is to make sure that the last sensor in a sequence of data transmissions — the one that’s actually going to send results to a human being’s laptop — has an accurate picture of what’s going on. “I’m not trying to get the right phone for me,” Dahleh says. “I just want you to get the right phone.”
According to Kleinberg, the new paper leaves a few salient questions unanswered, such as how quickly the network will converge on the correct answer, and what happens when the model of agents’ knowledge becomes more complex. But in several forthcoming papers, the MIT researchers begin to address both questions. One paper examines rate of convergence, although Dahleh and Acemoglu note that that its results are “somewhat weaker” than those about the conditions for convergence. Another paper examines cases in which different agents make different decisions given the same information: some people might prefer one type of cell phone, others another. In such cases, “if you know the percentage of people that are of one type, it’s enough — at least in certain networks — to guarantee learning,” Dahleh says. “I don’t need to know, for every individual, whether they’re for it or against it; I just need to know that one-third of the people are for it, and two-thirds are against it.” For instance, he says, if you notice that a Chinese restaurant in your neighborhood is always half-empty, and a nearby Indian restaurant is always crowded, then information about what percentages of people prefer Chinese or Indian food will tell you which restaurant, if either, is of above-average or below-average quality.
Reprinted with permission of MIT News.