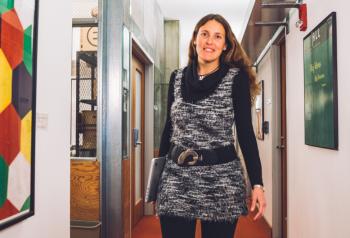
Photo: Lillie Paquette/MIT School of Engineering
Article Author
Date Published
March 24, 2016
Link to Original Article
Caroline Uhler joined the MIT faculty in October 2015 as an assistant professor in the Department of Electrical Engineering and Computer Science. She was awarded the 2015 Doherty Professorship in Ocean Utilization in November 2015. She joined the Institute for Data, Systems, and Society (IDSS) — which addresses complex societal challenges by advancing education and research at the intersection of statistics, data science, information and decision systems, and social sciences — as a member of the Laboratory for Information and Decision Systems (LIDS).
Uhler’s research focuses on mathematical statistics, in particular on graphical models and the use of algebraic and geometric methods in statistics, and its applications to biology. Her current projects include the development of causal inference algorithms to infer gene regulatory networks, the development of ellipsoid packing algorithms to study the spatial organization of chromosomes, and the study of Brownian motion models for phylogenetic inference using quantitative traits.
Uhler spoke with IDSS about her work and her perspective on being part of both LIDS and IDSS.
Q. How would you explain your work — both the theoretical and the applied — to someone not in the field?
A: Let’s start with graphical models. That’s my main interest. A graph in mathematical language is a network. So you have nodes [the points on the graph] and you have edges [the lines between the nodes].
There are two different kinds of models on a graph: Either you have data on the nodes or you have data on the edges. What I work on is gene expression data, so the nodes are the genes and the edges represent interactions between these genes. What we get to measure is the genes — how much protein they produce. Missing edges represent some kind of independence relation. Assuming you have knowledge on all nodes, a missing edge between gene 1 and gene 3 means that gene 1 does not provide any further information on gene 3 other than what we already know from all other genes.
The models I work on — with data on the nodes — can be undirected or directed. Undirected networks only represent association, meaning gene 1 has some effect on gene 2, but it carries no information about direct effect. In contrast, directed graphs can represent causal relationships, meaning that if this gene changes its expression by some amount, then the edge weights tell us by how much the expression of the other genes change. Most things in our world are directed — they have a cause-effect relationship. Such directed graphical models are therefore more informative and important for various applications. These are the kinds of models I am mainly working on.
Q. Genes are expressed in many ways: the color of your hair, the length of your bones. Are you interested in any particular trait or set of traits?
A: My interests are more basic. We have brain cells, and we have lung cells, and we have heart cells, and they are very different from each other. Even though gene expression varies a lot in different cell types, the DNA sequence in each one of our cells is approximately the same. So how is that possible? One thing I’m interested in is developing methods to infer gene regulatory networks for different cell types using causal graphs. Which are the key genes that are differentially expressed in the different cell types? What makes these differences?
Once the gene regulatory networks for the different cell types are known, the next challenge is to understand the mechanisms that drive the network structure. One of the hypotheses is that cell-type specific gene regulatory networks arise from differential packing of our genomes in the cell nucleus. Humans have 46 chromosomes and they’re all nicely packed as little ellipsoids into the nucleus. Interestingly, the nucleus in different cell types comes in different shapes, implying different packings of the chromosomes. Such packings allow for accessing different genes by the transcription factors — which are the [proteins] that turn on or off genes. These differences in packing could explain the emergence of different gene regulatory networks that lead to different cell types. I’m interested in modeling the spatial organization of the chromosomes using ellipsoid packing models to predict how the gene regulatory networks change in cellular differentiation and reprogramming.
Q. What was your path to academia?
A: My parents didn’t go to university. I think that made a big difference, since there were no expectations from their side about my career. I was always interested in teaching; so after high school I took one year off and I worked as a teacher at a secondary school. I taught German, Latin, French, and math. During that time, I realized that I liked teaching math the most. That’s how I decided to study math and take up undergraduate studies. I was always intrigued by the process of evolution, also, which led me to a minor in biology. In later years I got interested in statistics, since it’s the closest to biological applications and allowed me to combine my interests in math and biology. I met my future PhD advisor [Bernd Sturmfels] when he gave a course at [University of Zurich] called Algebraic Statistics in Computational Biology. It combined all areas that I loved: statistics, algebraic geometry, and biology. That’s how I ended up joining UC Berkeley as Professor Sturmfels’ PhD student.
Q. Why did you choose to join LIDS and IDSS?
A: LIDS fits very well with my interests in optimization and in graphical models. In LIDS there are faculty members with interests in similar areas, but with very distinct approaches. This allows for strong collaborations to develop and address important questions. This makes it very exciting for me.
IDSS is an extremely innovative and forward-looking venture and it’s just starting off. During my interviews I realized that IDSS was going to become truly interdisciplinary and I really enjoyed the very open and inclusive scientific culture that was present. I was certain that this was an ideal home to pursue my interdisciplinary research interests. Having been here the last few months, I can say that I am extremely happy to have decided to join MIT, and in particular LIDS and IDSS.
Reprinted with permission of MIT News.