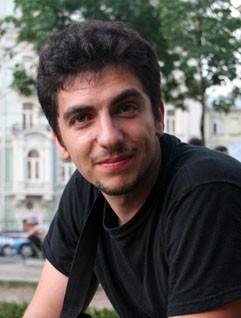
January 2, 2018
We are delighted to share that Professor Alexander (Sasha) Rakhlin, currently a Visiting Professor at LIDS, will be joining the MIT faculty through the Institute for Data, Systems, and Society (IDSS) and the Department of Brain and Cognitive Sciences (BCS), beginning January 15, 2018. He will also be joining LIDS as a Principal Investigator at that time.
We are proud to have a scholar of Sasha’s stature become a core member of our community. Please join us in extending him a warm welcome to LIDS!
Email Announcement to the IDSS Community
Dear Colleagues,
It is my pleasure to announce that Prof. Alexander (Sasha) Rakhlin has been appointed as an Associate Professor (with Tenure) at IDSS and BCS. Sasha’s appointment will start on Jan 15, 2018.
Before joining MIT, Sasha was an Associate Professor in the Department of Statistics at the University of Pennsylvania. He received his bachelor's degrees in mathematics and computer science from Cornell University, and a PhD in computational neuroscience from MIT. He was a postdoc at UC Berkeley EECS before joining UPenn, where he was a director of the Penn Research in Machine Learning center. He is a recipient of the NSF CAREER award, IBM Research Best Paper award, Machine Learning Journal award, and COLT Best Paper Award. He is currently an Associate Editor of the Annals of Statistics. He was on sabbatical at IDSS during 2016-2017. Sasha is a broad and highly accomplished scholar working at the interface of three fundamental data scientific fields: statistics, machine learning and optimization. This breadth of research has allowed him to take a unique vantage point and solve some of the most fundamental questions of data science by developing a mathematically deep and creative toolbox.
Approximately half of Sasha’s contributions focus on online learning; a framework pertinent to online decision-making, when the information is provided in a sequential fashion; a field that is now playing a preponderant role in machine learning due to the impetus of click-through-rate optimization in online advertising, or the role online learning plays in clinical trials as well as numerous other applications. A striking feature of modern online learning is that it relies on weak modeling assumptions and can make very precise predictions. This universality has explained the good practical performance of various ad hoc algorithms that were designed for this purpose. Such algorithms dominated the literature until a strong connection to the theory of convex optimization was uncovered, primarily in Sasha’s 2008 paper “Competing in the Dark” (with Abernethy and Hazan), which received two competitive best paper awards. A key insight of this work was leveraging the notion of self-concordance, a notion central to interior point methods in optimization, and showing that such a notion is critical in the context of learning as well. This work was followed by several influential papers that made the connection between learning and optimization even clearer through the lens of stochastic optimization. Sasha further revolutionized the field of online learning by providing a new theory around computational complexity and learnability. This contribution is at the level of the influential theory of Vapnik and Chervonenkis (VC theory) that has shaped learning theory over the past forty years. However, unlike VC theory, Sasha’s new theory gives unprecedented insights into the design of efficient algorithms, and does not rely on simplifying assumptions on the distribution of the data.
At the same time, Sasha has leveraged his expertise in machine learning to make fundamental contributions to statistics by developing new tools for optimal model selection. This is a critical and timely contribution as we live in an environment where many statistical methods are at our disposal. His “meta-method” allows for a combination of estimators in such a way to have the best generalization properties (beyond doing so over a cross-validation set). This combination does not correspond to simple linear combination and highlights the dependence of such construction on the underlying class of models.
Sasha has taught several classes in machine learning, statistical learning theory, probability theory, and optimization. More recently while visiting MIT, he taught the course “Online Methods in Machine Learning: Theory and Applications.” The course was a major hit by many of our students.
Please join me in welcoming Sasha to our faculty.
Best,
Munther Dahleh, Director, IDSS